Big Data
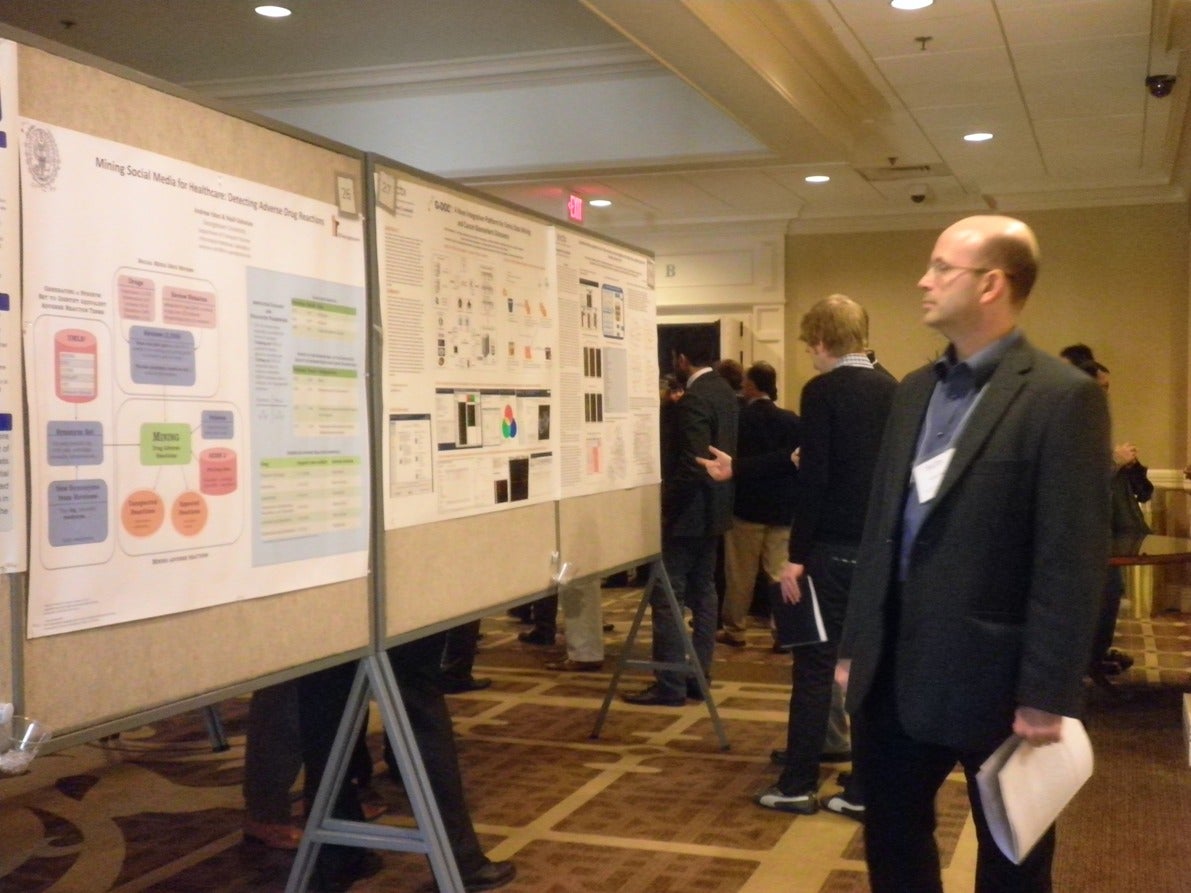
Practically every major sector of the economy and scientific enterprise is kindling (or rekindling) the idea of Big Data as key to solving important problems in and across disciplines. The Wall Street Journal has highlighted Big Data as one of three game changer or ‘black swan’ technologies that will transform the future. It is fair to say that there is a big data rush underway; nowhere is the promise and potential more real than in the rapid rise of inexpensive whole-genome sequencing technologies using next-generation sequencing (NGS) instruments. The world’s current sequencing capacity is estimated to be 13 quadrillion DNA bases a year. The cost to produce an accurate human whole-genome sequence is dropping rapidly and is expected to cost under $100 per genome in the next decade; the capacity to sequence the genomes of a billion people will have been realized in the next twenty years. These will be truly Big Data, requiring 3 or more exabytes of storage. A number of public and private projects are already contributing to this biological data deluge. The NIH-funded 1000 Genomes Project deposited 200 terabytes of raw sequencing data into the GenBank archive during the project’s first 6 months of operation, twice as much as had been deposited into all of GenBank for the entire 30 years preceding.
Our team is working on a new generation of data management and mining systems to support diverse needs from personalized clinical medicine, translational and population research involving molecular and genetic outcomes. This project spans the disciplines of computer systems, analytics, and genomics. Our systems research, in collaboration with the departments of computer science at Virginia Tech and Georgetown University will help harness petabytes of genomic information in a manner cognizant of the multimodal, multilevel nature of the datasets, and our project will extend the query capabilities of existing NoSQL models. Our analytics research extends the MapReduce workflow paradigm to support more complex workflows on genomic and other biological data while being attentive to the need to save some intermediary results and discard others. More broadly, this project is aimed to be the first implementation of petabyte-scale compositional data mining to extract actionable, ranked knowledge from large-scale genome studies.