Robert Beckman, MD
Professor
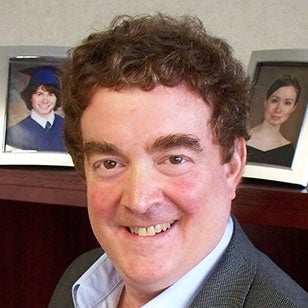
Robert A. Beckman, M.D., an oncology clinical researcher and mathematical biologist, has played significant leadership roles in developing new oncology clinical research groups at 4 pharmaceutical companies and in 5 cross-company collaborations, and brought 23 new oncology therapies into man, and 2 to market.. He has co-invented novel clinical study designs and development strategies, and leads a 200 person international group in this field. Dr. Beckman’s theoretical studies of cancer evolution predicted broad features of tumor evolution before experimental results were available, and have more recently led to a new approach to cancer precision medicine, dynamic precision medicine, which holds promise for significant improvement in patient outcomes. Dr. Beckman has a BA in Biochemical Sciences from Harvard College and an MD from Harvard Medical School, and completed clinical training in pediatrics (Stanford University) and pediatric oncology (University of Michigan), and postdoctoral work at Fox Chase Cancer Center, the Bristol-Myers Squibb Pharmaceutical Research Institute, and the University of Michigan, the latter under an NIH Physician-Scientist Award. He has served on the University of Michigan Biophysics faculty, been a Visiting Scientist within the Warner Lambert/Park Davis Biomolecular Structure and Drug Design Group (1994-1995), and a Member/Visitor in Systems Biology at the Institute for Advanced Study, Princeton, New Jersey (2008-2009). His versatile publication record comprises over 280 contributions ranging from computational chemistry to clinical oncology, emphasizing quantitative approaches. He is currently Professor of Oncology and of Biostatistics, Bioinformatics, and Biomathematics at Lombardi Comprehensive Cancer Center and the Innovation Center of Biomedical Informatics, Georgetown University Medical Center and Scientific Advisor to the Senior Vice President for Research, Georgetown University.
View a complete list of his publications on Google Scholar.
Recent Publications
Other Key Publications
Adaptive design for a confirmatory basket trial in multiple tumor types based on a putative predictive biomarker RA Beckman, Z Antonijevic, R Kalamegham, C Chen. Clinical Pharmacology and Therapeutics 100, 617-625, 2016.
Phase I imaging and pharmacodynamics trial of CS-1008 in patients with metastatic colorectal cancer. M Ciprotti, NC Tebbutt, FT Lee, ST Lee, HK Gan, DC McKee, GJ O’Keefe, SJ Gong, G Chong, W Hopkins, W Hopkins, FE Scott, MW Brechbiel, AN Tse, M Jansen, M Matsmura, M Kotsuma, R Watanabe, R Venhaus, RA Beckman, J Greenberg, AM Scott. Journal of Clinical Oncology 33, 2609-2616, 2015.
Impact of genetic dynamics and single-cell heterogeneity on development of nonstandard personalized medicine strategies for cancer. RA Beckman, GS Schemmann, CH Yeang. Proc. Natl. Academy of Sciences USA 109, 14586-14591, 2012.
Phase I pharmacologic and pharmacodynamic study of the gamma secretase (Notch) inhibitor MK-0752. I Krop, T Demuth, T Guthrie, PY Wen, W Mason, P Chinnayan, N Butowksi, MD Groves, S Kesari, S Blackman, J Watters, A Loboda, A Podtelezhnikov, J Luceford, C Chen, M Giannotti, J Hing, RA Beckman, P LoRusso. Journal of Clinical Oncology 30, 2307-2313, 2012.
Integrating predictive biomarkers and classifiers into oncology clinical development programmes. RA Beckman, J Clark, C Chen. Nature Reviews Drug Discovery 10, 735-749, 2006.
Antibody constructs in cancer therapy: protein engineering strategies to improve exposure in solid tumors. RA Beckman, LM Weiner, H Davis. Cancer 109, 170-179, 2007.
Efficiency of carcinogenesis without a mutator mutation RA Beckman, LA Loeb. Proc. Natl. Academy of Sciences USA 103: 14140-14145, 2006.
Mathematical Modeling of Tumor Evolution, Intratumoral Heterogeneity, and Dynamic Precision Medicine
While current precision medicine customizes therapy based on molecular differences between patients, there are extensive differences in cancer within single patients between individual cancer cells. Moreover, current precision medicine does not systematically take account of the fact that the molecular characteristics of a cancer are not static, but evolving. Intratumoral heterogeneity and tumor evolutionary dynamics are sources of therapeutic resistance, and limit the effectiveness of current precision medicine protocols. We are involved in the following projects:
Extensive Subclonal Diversity in Colorectal Cancer: using an extremely accurate technique termed duplex DNA sequencing, we have explored subclonal heterogeneity at higher DNA sequencing depth than was previously possible, allowing us to detect rare subclonal variations. We have developed new theoretical methods that are more applicable for cell populations as are found in clinical tumors. Using these new methods, we have determined that diversity is far greater than previously anticipated, and that, based on the data and a quantitative evolutionary model, every DNA nucleotide is mutated in at least one cell in the smallest tumor visible on CT, unless that mutation is highly selected against. This means that there is likely to be at least one cell which is resistant to any single therapy pre-existing at diagnosis. However, based on our quantitative model, cells that are simultaneously resistant to multiple therapies are not likely to be present at diagnosis, but rather evolve from singly resistant cells during therapy and subsequent tumor growth and evolution.
Comprehensive Characterization of Subclonal Diversity in Tumors: previous work characterizing subclonal diversity has been dependent on the depth of DNA sequencing. With variable shallow depth of sequencing, the field has been characterizing diversity in an analogous manner to quantifying icebergs by what is visible above the surface. In addition to sequencing more deeply than previously, we have developed a mathematical approach to comprehensive characterization of diversity within the tumor by sequencing at multiple depths. The model predicts the experimental data with correlation coefficients above 0.95 and enables prediction of the total diversity that would have been obtained if each of the billions of cells in the tumor had been sequenced individually.
Neutral evolution of tumors: while selection has an important role to play in the initial development of tumors, allowing them to acquire key oncogenic mutations, we hypothesized that random, unselected “neutral” evolution would lead to a reservoir of pre-existing diversity which could be a source of drug resistance. We demonstrated mathematically that the branching nature of tumor evolution creates rarer subclones at later timepoints, and that sequencing a single sample at multiple depths (which can in turn detect rarer subclones) therefore provides a cross section through time. Using this approach and the data from the diversity of colorectal cancer, we have shown that most of the diversity within colorectal cancer is occurring at neutral sites not subject to selection prior to therapy. The diversity in these sites may be critical to resistance and thus be selected by therapy itself.
Dynamic precision medicine: current precision medicine does not systematically take into account extensive intratumoral heterogeneity nor evolutionary dynamics, nor does it plan ahead. Rather it matches therapies to static patterns, one step at a time. We have developed dynamic precision medicine, a method for planning individualized treatment sequences taking heterogeneity and evolutionary dynamics into account. Simulations show that this approach can in principle lead to highly significant improvements in survival and cure rates among metastatic cancer patients.
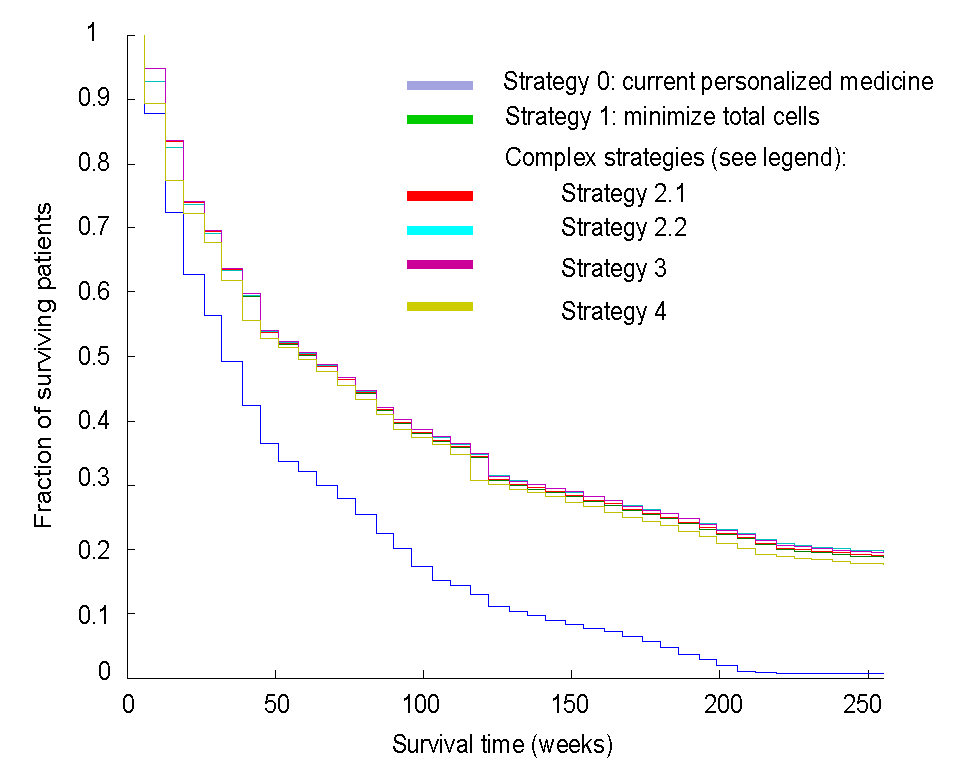
In a comprehensive simulation of 3 million virtual oncology patients, designed to mirror possible presentations throughout oncology, dynamic precision medicine significantly outperformed conventional precision medicine (blue line)
Hypermutator cells in tumor evolution: the above predicts that every tumor at diagnosis will contain at least one cell that is resistant to any therapeutic agent. However, it also predicts that in many cases further tumor growth and evolution during therapy will be required for cells to arise that are simultaneously resistant to multiple non-cross resistant therapies. Further, the above predicts that a subset of the tumor cells will have additional mutations in DNA replication repair and machinery, increasing their mutation rate and speeding their evolution, and that these “hypermutator cells” may be critical therapeutic targets. We are developing assays for identifying and isolating hypermutator cells using fluorescent cell sorting (FACS) after transfection with two mutant chromophore genes which require mutations to “light up”. Isolated hypermutator cells might be subjected to high throughput drug sensitivity screening and influence the therapeutic plan under dynamic precision medicine. Funded by contract with Oak Ridge National Laboratories.
Dynamic precision medicine in HER2+-ER+ (double positive breast cancer): in this uncommon type of breast cancer, agents normally effective against HER2+ breast cancer and ER+ breast cancer are not effective either individually or in combination. We are characterizing this condition at a single cell level, and hypothesize that the condition may be driven by hypermutator cells. Ultimately we hope to develop a model of dynamic precision medicine specific to this condition
Optimal dynamic treatment sequences incorporating non-genetic heterogeneity/plasticity: we are studying feedback loops involving b-raf and NKB signaling pathways in melanoma. Modeling indicates periodic application of therapy in an on-off cycle optimizes results with respect to short term reversible phenomena like feedback loops.
Clinical trial designs for dynamic precision medicine: testing dynamic precision medicine may be challenging in that it specifies very frequent individualized therapy adaptations (as often as every six weeks) based on evolutionary dynamics. A new clinical trial design, the evolutionary hyperbasket trial, has been developed and its performance is undergoing simulation studies. The design may be useful for other evolutionary approaches to cancer medicine.
Clinical Trial Design
Clinical trials in oncology consume the largest proportion of resources in oncology today, and the cost of discovering and validating a new effective oncology agent and associated biomarkers averages approximately $1 billion dollars. Increasing molecular biology knowledge is leading to an explosion of possible clinical hypotheses deserving testing, many of them for small subgroups of patients defined by molecular biomarkers. These subgroups pose a challenge for enrollment. Biomarkers and their associated assays require validation for clinical utility and cost effectiveness. We are involved in the following projects:
Drug Information Association (DIA) Adaptive Design Scientific Working Group: We provide leadership for this group of over 200 statisticians and clinicians from the Food and Drug Administration (FDA) and European Medicines Agency (EMA), academics, and the pharmaceutical and biotechnology industry, which is interested in developing improved clinical trial designs for both rare diseases and small biomarker-defined subgroups of large diseases like cancer. We are involved in the following projects:
Confirmatory Basket Trials: Basket trials are a new trial design in which cancers from different organ sites are pooled into the same trial based on shared molecular features. Most basket trials to date have been either at the exploratory phase of drug development, or for therapies with an unusual level of effectiveness warranting aggressive development approaches. The group has developed a general design which will be useful for confirmatory designs leading to approval of therapies for clinical use, including the majority of potential therapies, not only a few outstanding ones. The confirmatory space is where the vast majority of drug development resources are currently spent, positioning this development as one that has the potential to greatly reduce drug development costs. We were one of three clinical trial design expert groups invited from the US to advise the EMA on basket trial designs, and have presented extensively at national meetings, and at academic and commercial venues.
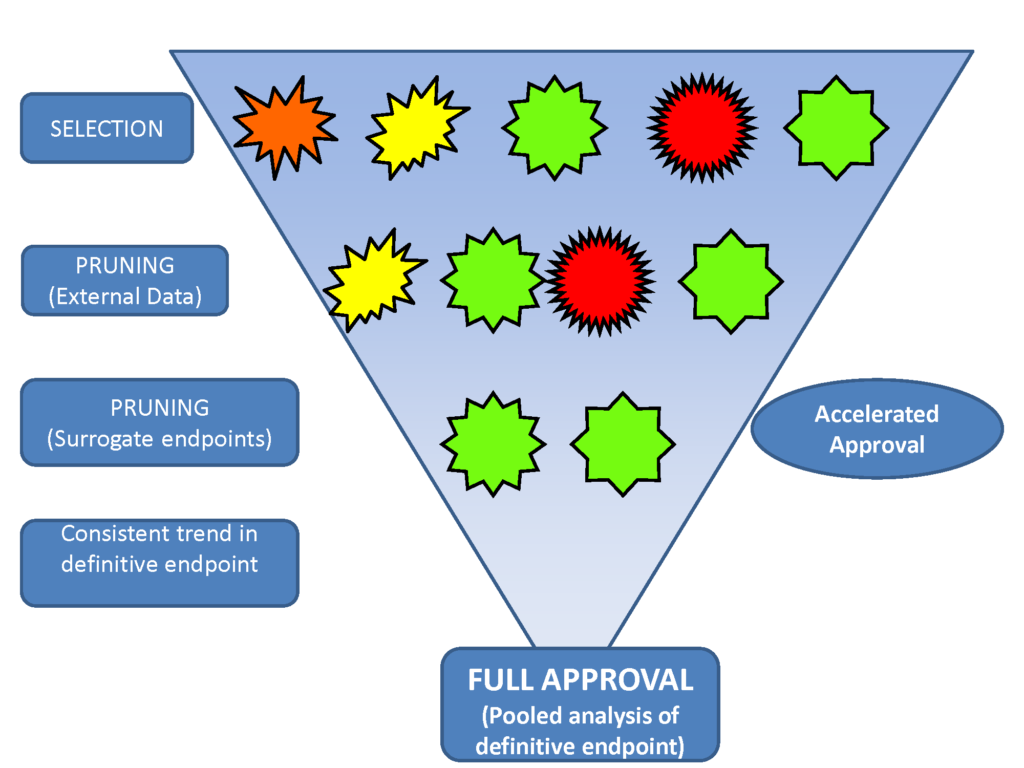
High Level Design of Confirmatory Basket Trial
(shapes represent indications)
Application of real world data (RWD) to design of confirmatory basket trials: Using RWD from MEDSTAR/Georgetown electronic health records, we are designing and simulating a real world basket trial among current off-label indications of rituximab in auto-immune disorders. The indications and endpoints are selected with literature data alone, and with literature data + RWD, and the performance of the basket trial will be compared under these conditions.
PIPELINES: We were involved in a publication together with several national and international leaders in the clinical trial space discussing the possibilities inherent in linking platform trials into a national or worldwide system.
Informational Adaptive Design: adaptation in oncology often occurs early in a trial based on endpoints whose relationship to benefit endpoints like survival is uncertain. This project has developed alternative methods for adaptations which may be postponed until the end of the trial. A subset of the information using definitive endpoints is used to inform these decisions, like a Phase 2 trial embedded in a Phase 3. This has been applied to subgroup selection, and we are currently exploring endpoint selection, an approach which may have great application in rare diseases, where current FDA guidance often recommends non-therapeutic “natural history” studies to inform endpoint selection in therapeutic studies. The informational design may replace natural history studies under some rare disease circumstances, hastening initiation of therapeutic studies in these often serious and life-threatening conditions
Adaptive Alpha Allocation: Often in personalized medicine we do not know upfront whether a therapy will be effective in the full population or in a biomarker defined subset. Methods have been developed to test both possibilities at once optimally, while controlling the rate of false positive discovery (alpha).
Predictive Biomarker Subgroup Methodologies Utilizing Decision Analysis from Multiple Perspectives: therapy and biomarker development in personalized medicine require decisions of when to proceed with phase 3 in the full population versus a subpopulation versus an adaptive design, optimal sample sizes, and whether to proceed at all, among others. Decision analysis allows determination of optimal development pathways from the drug developer’s perspective, the health authority perspective, and the public health perspective. We are currently following up on situations where incentives may be misaligned between these stakeholders.
Extent of evidence needed for decisions in rare diseases: Rare diseases may not have enough patients to permit clinical trials with standard designs and endpoints for confirmation of effectiveness and marketing approval. This research work is exploring novel development pathways in these challenging situations, particularly defining more rigorous approaches for use of historical controls.
Master Protocols: master protocols include basket trials (above) and umbrella trials which match patients in multiple biomarker subgroups to multiple therapies. Ongoing work is investigating when different types of designs are best used and how to make decisions concerning patients who might belong to more than one overlapping subgroup. A book, Platform Trials in Drug Development: Umbrella Trials and Basket Trials, was published by Chapman and Hall/CRC Press in 2018.
2-stage Proof of Concept Arrays: Some drug developers prefer not to spend available resources for proof of concept trials simultaneously, but rather invest in a small number and continue with additional studies for a given drug only if the small number of trials produces a success. This improves cost effectiveness at the expense of lowering throughput. We have developed a quantitative model for determining when this strategy is advantageous.
Type III error: Previously, statisticians have focused mainly on minimizing type I error (false positives) and type II error (false negatives). Yet in a resource constrained environment there is a tradeoff between these parameters and cost. We have defined “Type III error”, which is the opportunity cost when a trial that would have led to a successful therapy was not performed due to lack of funds, and researched optimal tradeoffs between the three types of error in oncology development scenarios.